Please download and install the prolfquadata
package
from github
Load data
We start by loading the IonStar dataset and the annotation from the
prolfquadata
package. The method inner_join
adds the annotation to the data.
datadir <- file.path(find.package("prolfquadata") , "quantdata")
inputFragfile <- file.path(datadir, "MSFragger_IonStar2018_PXD003881.zip")
inputAnnotation <- file.path(datadir, "annotation_Ionstar2018_PXD003881.xlsx")
annotation <- readxl::read_xlsx(inputAnnotation)
## Error in get(paste0(generic, ".", class), envir = get_method_env()) :
## object 'type_sum.accel' not found
Create prolfqua configuration
atable <- prolfqua::AnalysisTableAnnotation$new()
atable$fileName = "raw.file"
atable$hierarchy[["protein_Id"]] <- c("protein")
atable$hierarchyDepth <- 1
atable$set_response("razor.intensity")
atable$factors[["dilution."]] = "sample"
atable$factors[["run"]] = "run_ID"
atable$factorDepth <- 1
config <- prolfqua::AnalysisConfiguration$new(atable)
adata <- prolfqua::setup_analysis(merged, config)
lfqdata <- prolfqua::LFQData$new(adata, config)
lfqdata$remove_small_intensities()
Normalize data using human proteins
pl <- lfqdata$get_Plotter()
pl$intensity_distribution_density()
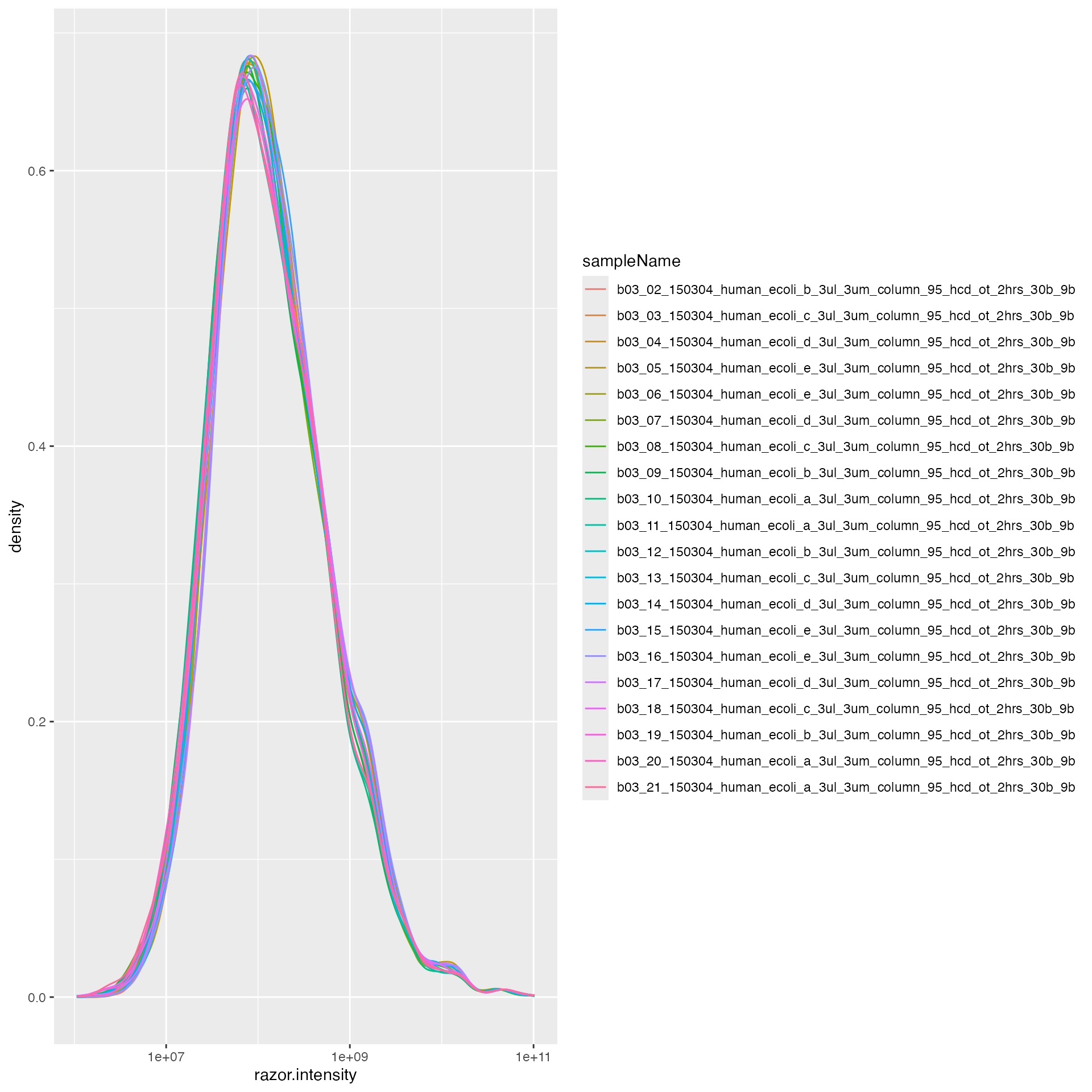
subset_h <- lfqdata$get_copy()$get_Transformer()$log2()$lfq
subset_h$data <- subset_h$data |> dplyr::filter(grepl("HUMAN", protein_Id))
tr <- lfqdata$get_Transformer()
lfqdataNormalized <- tr$log2()$robscale_subset(lfqsubset = subset_h)$lfq
pl <- lfqdataNormalized$get_Plotter()
pl$intensity_distribution_density()
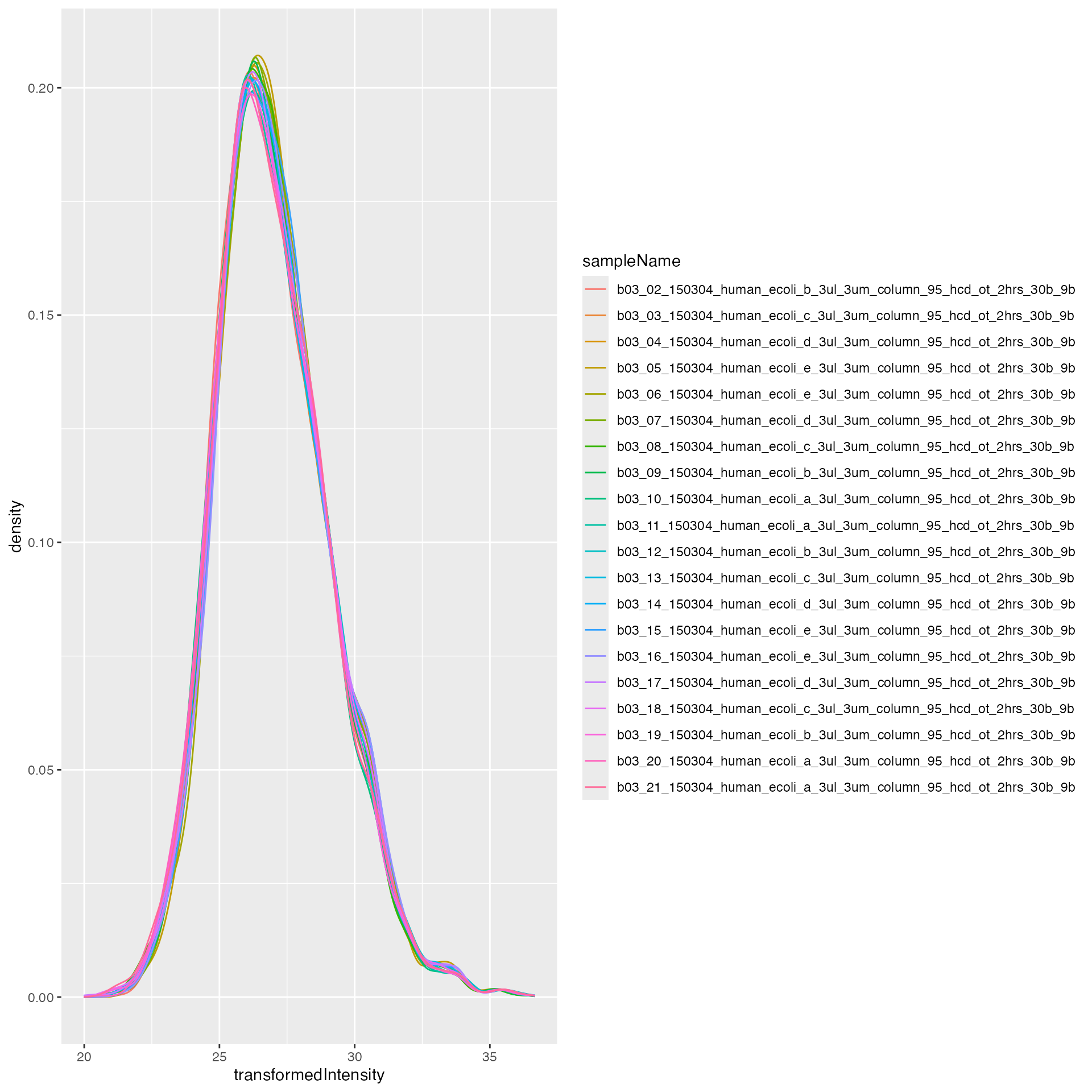
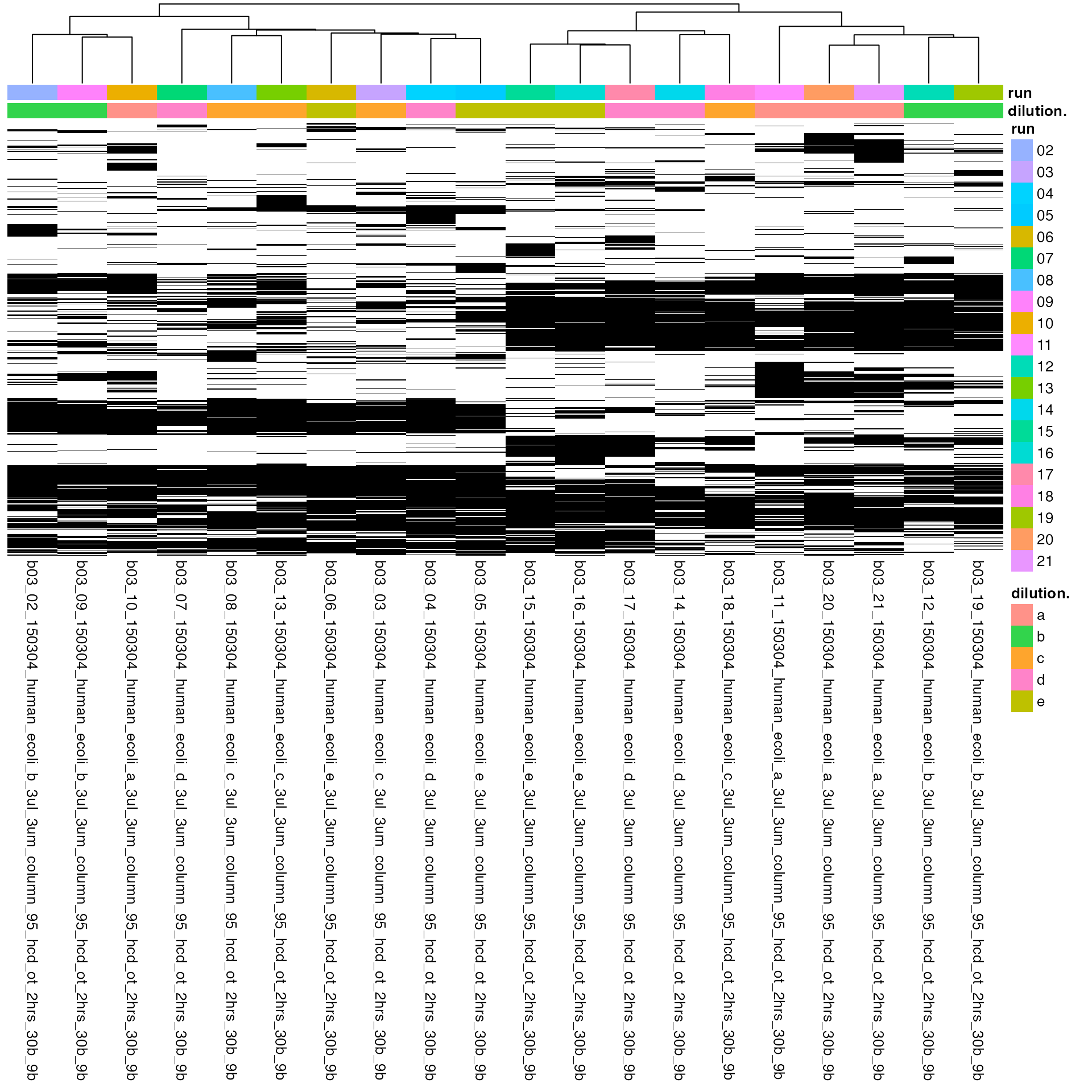
Model data using prolfqua and specify contrasts
Contrasts <- c(
"dilution_(9/7.5)_1.2" = "dilution.e - dilution.d",
"dilution_(7.5/6)_1.25" = "dilution.d - dilution.c",
"dilution_(6/4.5)_1.3(3)" = "dilution.c - dilution.b",
"dilution_(4.5/3)_1.5" = "dilution.b - dilution.a"
)
lmmodel <- "~ dilution."
lmmodel <- paste0(lfqdataNormalized$config$table$get_response() , lmmodel)
modelFunction <- prolfqua::strategy_lm( lmmodel, model_name = "Model")
mod <- prolfqua::build_model(lfqdataNormalized$data, modelFunction)
contr <- prolfqua::Contrasts$new(mod, Contrasts)
contrimp <- prolfqua::ContrastsMissing$new(lfqdataNormalized, Contrasts)
merged <- prolfqua::merge_contrasts_results(contr, contrimp)
mergedmod <- prolfqua::ContrastsModerated$new(merged$merged)
cp <- mergedmod$get_Plotter()
cp$volcano()
## $FDR
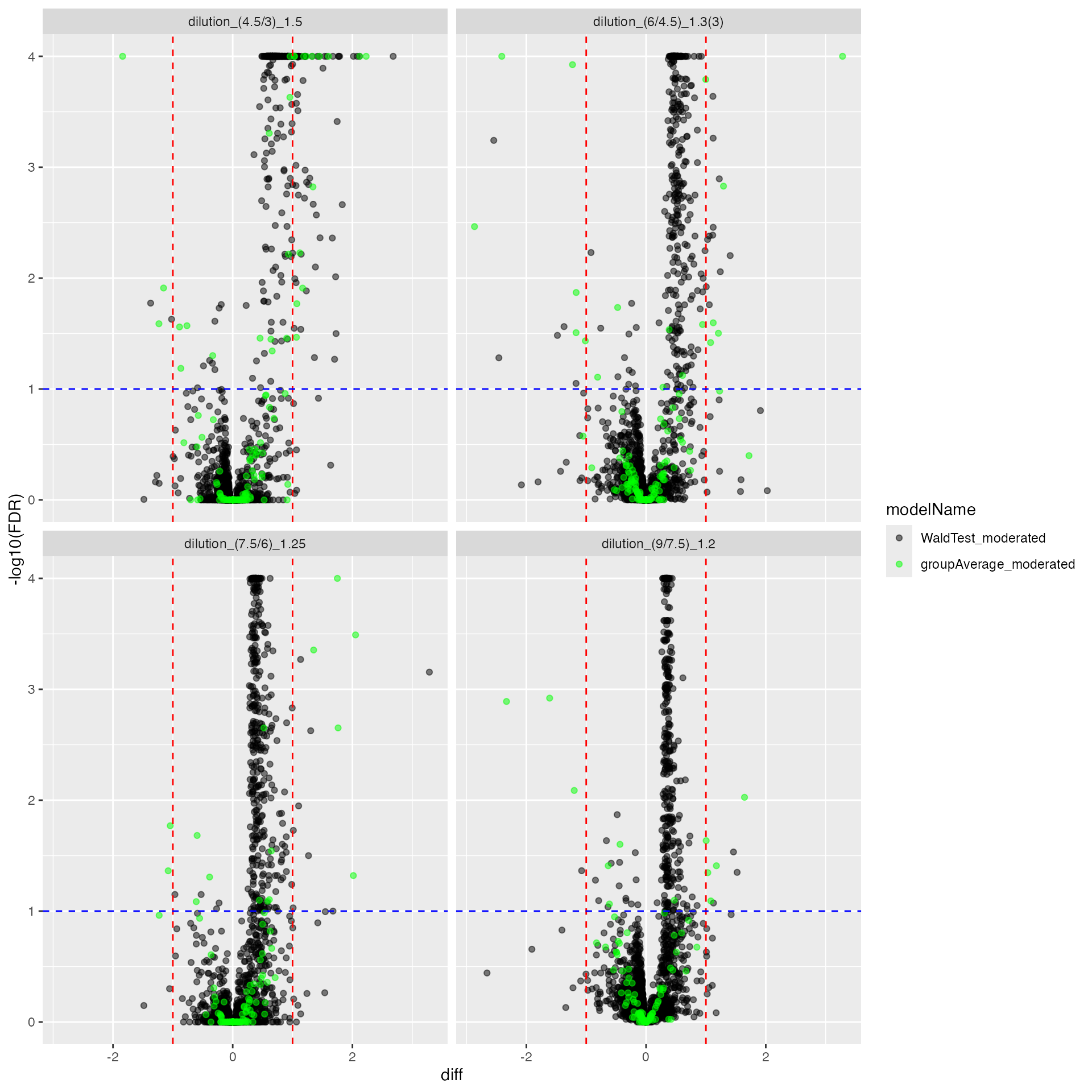
Benchmark data
ttd <- prolfqua::ionstar_bench_preprocess(mergedmod$get_contrasts())
benchmark_prolfqua <- prolfqua::make_benchmark(ttd$data,
model_description = "MSFragger med. polish and lm. density",
model_name = "MSFragger_prot_med_lm",
FDRvsFDP = list(list(score = "FDR", desc = FALSE))
)
knitr::kable(benchmark_prolfqua$pAUC_summaries()$ftable$content)
all |
diff |
94.03414 |
72.03297 |
80.81840 |
all |
scaled.p.value |
95.12016 |
81.86899 |
85.01266 |
all |
statistic |
95.01501 |
80.86869 |
84.54175 |
dilution_(4.5/3)_1.5 |
diff |
91.74353 |
71.35642 |
74.94692 |
dilution_(4.5/3)_1.5 |
scaled.p.value |
91.97449 |
74.07253 |
75.69785 |
dilution_(4.5/3)_1.5 |
statistic |
91.88659 |
73.16519 |
75.26106 |
dilution_(6/4.5)_1.3(3) |
diff |
94.51047 |
77.29426 |
83.11132 |
dilution_(6/4.5)_1.3(3) |
scaled.p.value |
95.25739 |
82.86520 |
85.39251 |
dilution_(6/4.5)_1.3(3) |
statistic |
95.15824 |
82.03018 |
84.98705 |
dilution_(7.5/6)_1.25 |
diff |
93.58321 |
64.35484 |
79.10116 |
dilution_(7.5/6)_1.25 |
scaled.p.value |
95.02455 |
82.23264 |
86.55908 |
dilution_(7.5/6)_1.25 |
statistic |
94.93966 |
81.16409 |
86.10408 |
dilution_(9/7.5)_1.2 |
diff |
95.95465 |
74.05003 |
85.26557 |
dilution_(9/7.5)_1.2 |
scaled.p.value |
97.96069 |
89.12013 |
92.60967 |
dilution_(9/7.5)_1.2 |
statistic |
97.80569 |
87.74253 |
91.93801 |
prolfqua::table_facade(benchmark_prolfqua$smc$summary, "Nr of estimated contrasts")
Nr of estimated contrasts
0 |
3836 |
benchmark_prolfqua$plot_score_distribution()
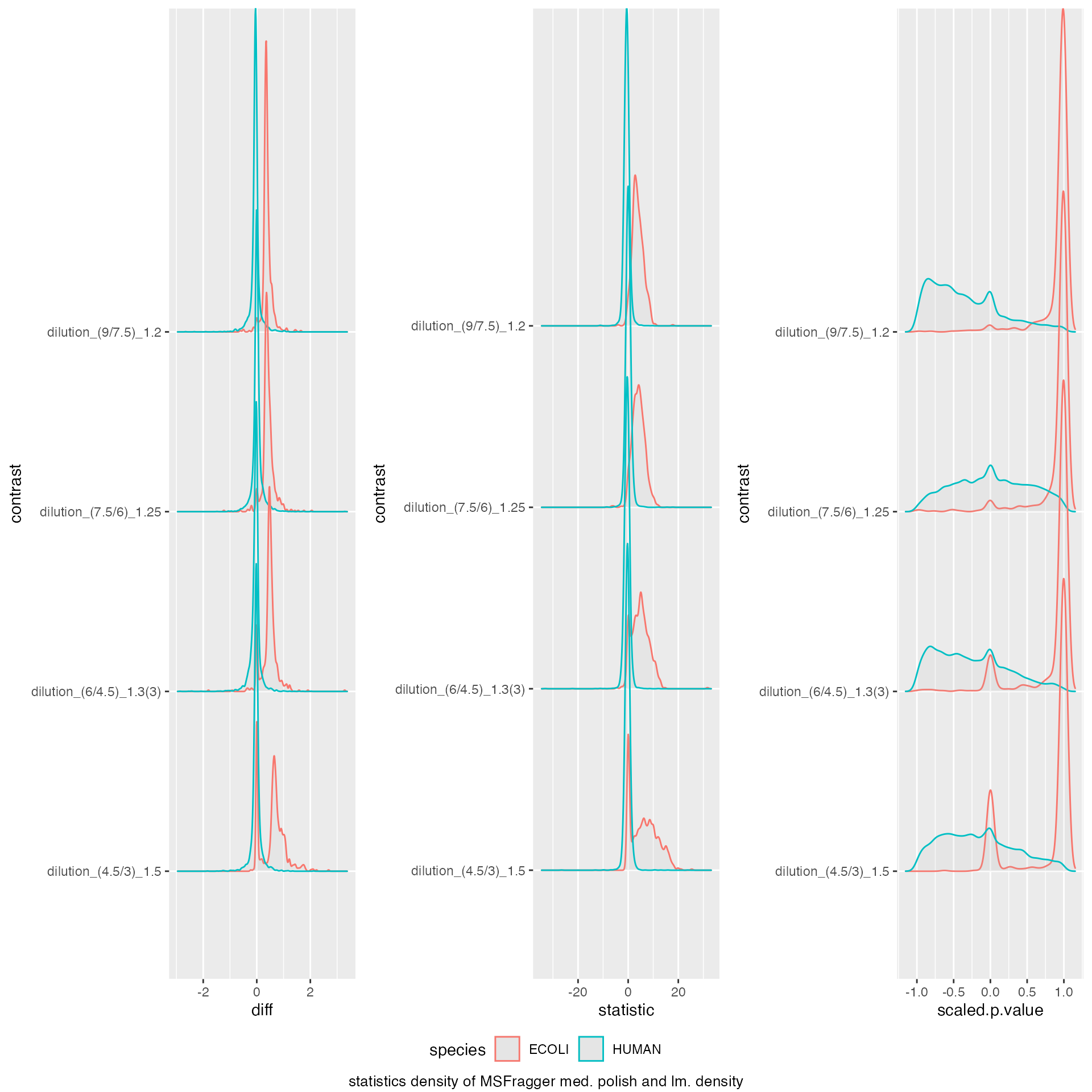
benchmark_prolfqua$plot_ROC(0.05)
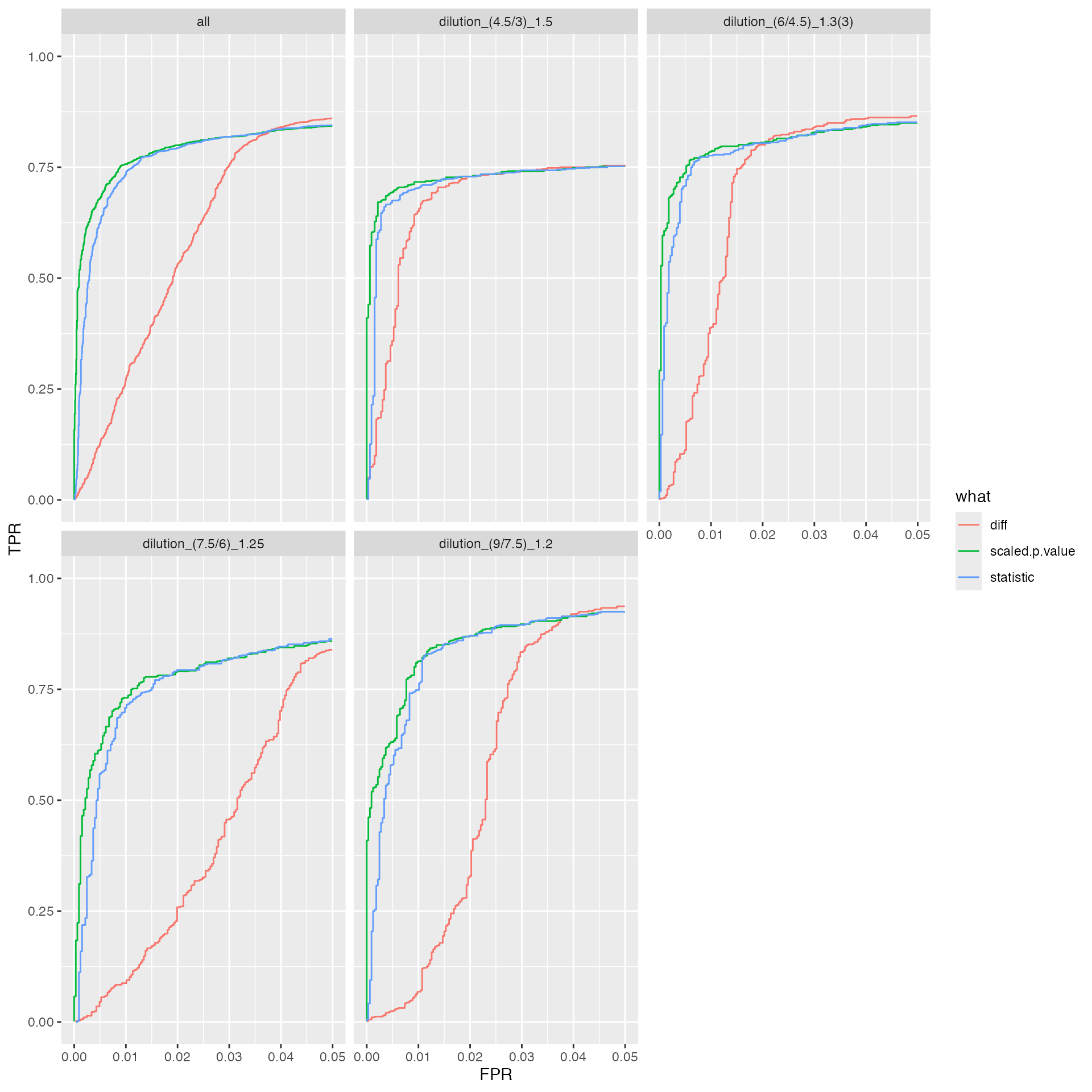
benchmark_prolfqua$plot_FDRvsFDP()
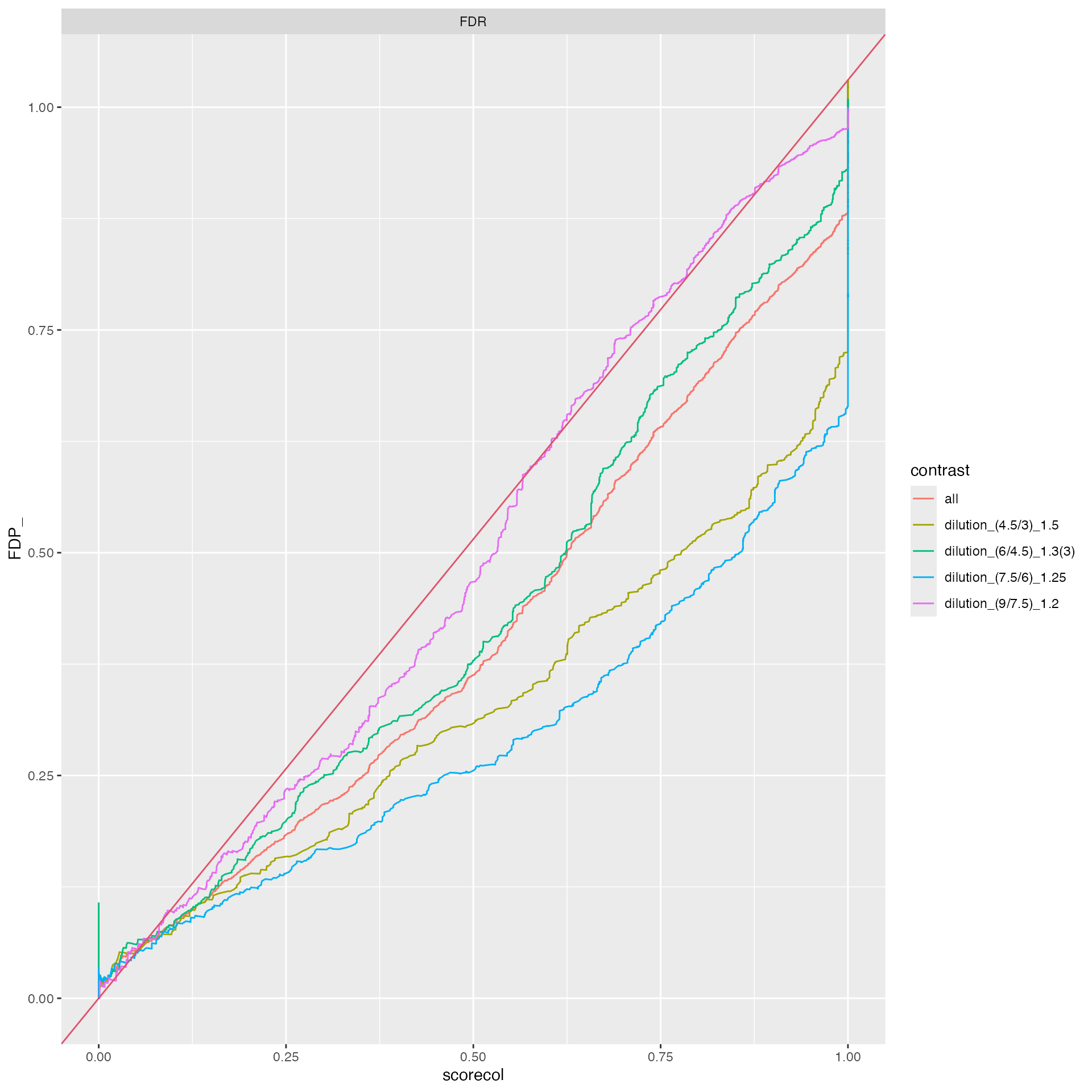
Model data using proDA and specify contrasts
se <- prolfqua::LFQDataToSummarizedExperiment(lfqdataNormalized)
fit <- proDA::proDA(se, design = ~ dilution. - 1, data_is_log_transformed = TRUE)
contr <- list()
contr[["dilution_(9/7.5)_1.2"]] <- data.frame(
contrast = "dilution_(9/7.5)_1.2",
proDA::test_diff(fit, contrast = "dilution.e - dilution.d"))
contr[["dilution_(7.5/6)_1.25"]] <- data.frame(
contrast = "dilution_(7.5/6)_1.25",
proDA::test_diff(fit, contrast = "dilution.d - dilution.c"))
contr[["dilution_(6/4.5)_1.3(3)"]] <- data.frame(
contrast = "dilution_(6/4.5)_1.3(3)",
proDA::test_diff(fit, contrast = "dilution.c - dilution.b"))
contr[["dilution_(4.5/3)_1.5"]] <- data.frame(
contrast = "dilution_(4.5/3)_1.5",
proDA::test_diff(fit, contrast = "dilution.b - dilution.a" ))
bb <- dplyr::bind_rows(contr)
Benchmark data
ttd <- prolfqua::ionstar_bench_preprocess( bb , idcol = "name" )
benchmark_proDA <- prolfqua::make_benchmark(ttd$data,
contrast = "contrast",
toscale = c("pval"),
fcestimate = "diff",
benchmark = list(
list(score = "diff", desc = TRUE),
list(score = "t_statistic", desc = TRUE),
list(score = "scaled.pval", desc = TRUE)
),
model_description = "proDA_medpolishInt",
model_name = "proDA_medpolishInt",
FDRvsFDP = list(list(score = "adj_pval", desc = FALSE))
, hierarchy = c("name"), summarizeNA = "t_statistic"
)
sumarry <- benchmark_proDA$smc$summary
prolfqua::table_facade(sumarry, caption = "nr of proteins with 0, 1, 2, 3 missing contrasts.")
nr of proteins with 0, 1, 2, 3 missing contrasts.
0 |
3836 |
knitr::kable(benchmark_proDA$pAUC_summaries()$ftable$content)
all |
diff |
91.99592 |
61.86335 |
76.45600 |
all |
scaled.pval |
94.25411 |
80.02409 |
83.62640 |
all |
t_statistic |
94.25411 |
80.02409 |
83.62640 |
dilution_(4.5/3)_1.5 |
diff |
89.74745 |
69.12772 |
76.47584 |
dilution_(4.5/3)_1.5 |
scaled.pval |
91.77920 |
74.78168 |
77.29185 |
dilution_(4.5/3)_1.5 |
t_statistic |
91.77920 |
74.78168 |
77.29185 |
dilution_(6/4.5)_1.3(3) |
diff |
92.40180 |
68.82831 |
79.75247 |
dilution_(6/4.5)_1.3(3) |
scaled.pval |
94.09976 |
81.69756 |
84.47232 |
dilution_(6/4.5)_1.3(3) |
t_statistic |
94.09976 |
81.69756 |
84.47232 |
dilution_(7.5/6)_1.25 |
diff |
91.19929 |
48.04371 |
70.53692 |
dilution_(7.5/6)_1.25 |
scaled.pval |
93.94111 |
79.59794 |
84.24361 |
dilution_(7.5/6)_1.25 |
t_statistic |
93.94111 |
79.59794 |
84.24361 |
dilution_(9/7.5)_1.2 |
diff |
94.43666 |
61.61623 |
79.02591 |
dilution_(9/7.5)_1.2 |
scaled.pval |
97.21442 |
85.21924 |
89.29099 |
dilution_(9/7.5)_1.2 |
t_statistic |
97.21442 |
85.21924 |
89.29099 |
prolfqua::table_facade(benchmark_prolfqua$smc$summary, "Nr of estimated contrasts")
Nr of estimated contrasts
0 |
3836 |
benchmark_proDA$plot_score_distribution()
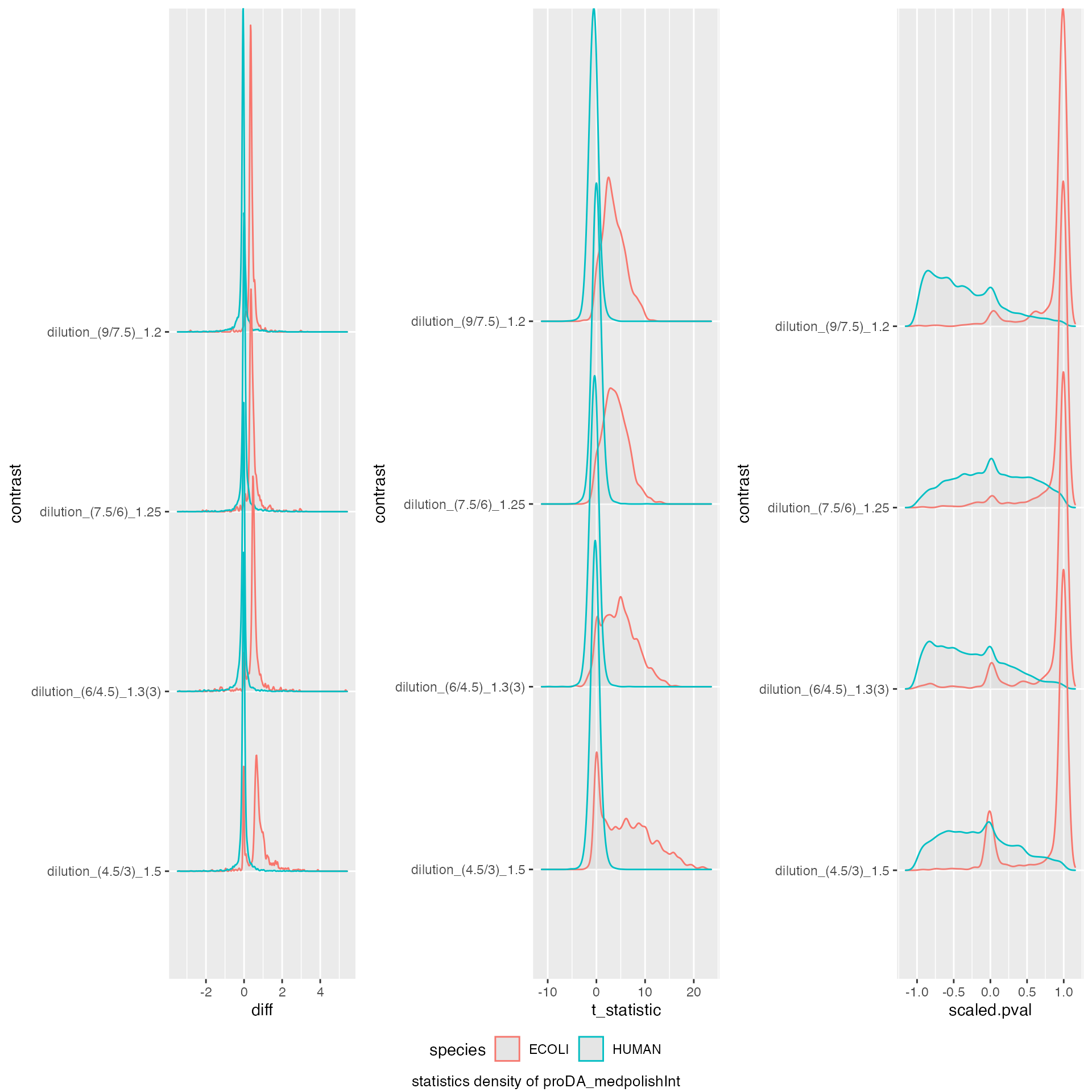
benchmark_proDA$plot_ROC(0.05)
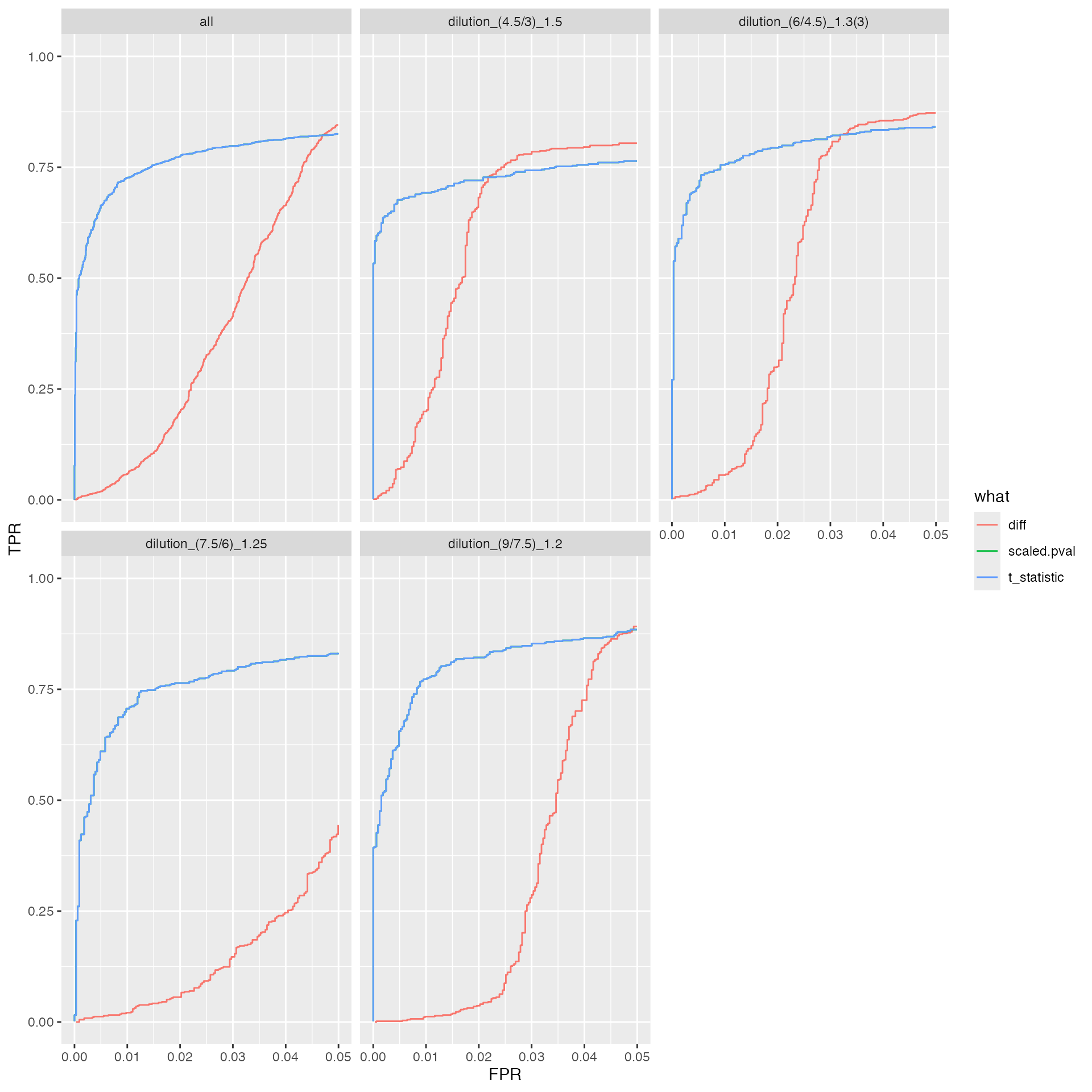
benchmark_proDA$plot_FDRvsFDP()
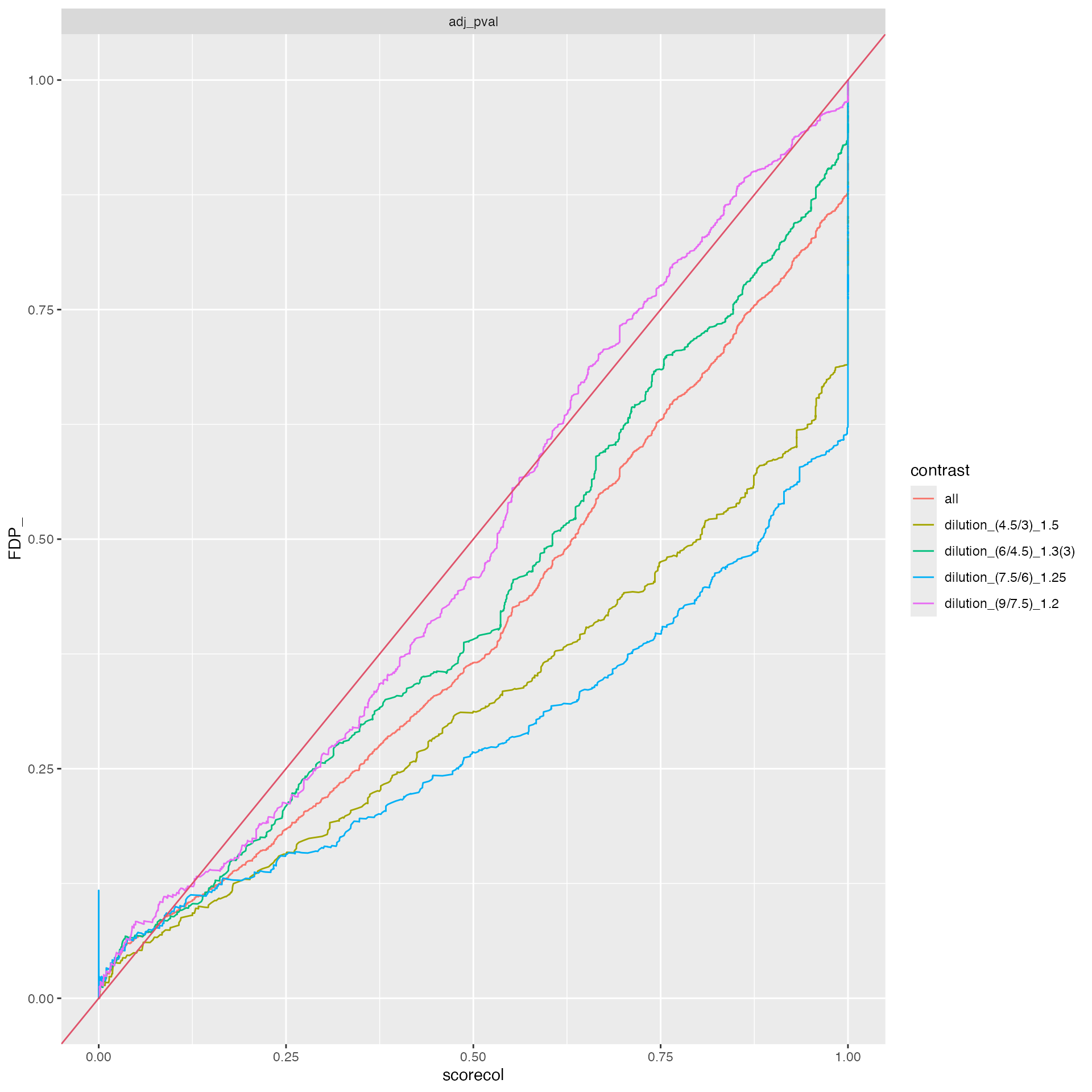
Compare prolfqua and proda
Direct comparison with msqrob2 is impossible since, to fit the
dropout model, the peptide intensities are required, while here, we are
starting the analysis from the combined_proteins.tsv
file.
bdir <- file.path("../inst/Benchresults/",format( Sys.Date(), "%Y%m%d"))
if (!dir.exists(bdir)) {dir.create(bdir)}
saveRDS(list(benchmark_proDA = benchmark_proDA, benchmark_prolfqua = benchmark_prolfqua)
,file.path("../inst/Benchresults/",format( Sys.Date(), "%Y%m%d"),"FragPipev14_comb_prot.RDS"))
proda <- benchmark_proDA$pAUC_summaries()$ftable$content
proda$package <- "proda"
prolfqua <- benchmark_prolfqua$pAUC_summaries()$ftable$content
prolfqua$package <- "prolfqua"
tmp <- dplyr::bind_rows(proda, prolfqua)
tmp$what |> unique()
## [1] "diff" "scaled.pval" "t_statistic" "scaled.p.value"
## [5] "statistic"
tmp$what[tmp$what == "statistic"] <- "t_statistic"
tmp$what[tmp$what == "scaled.pval"] <- "scaled.p.value"
tmp |> ggplot2::ggplot(ggplot2::aes(x = what, y = pAUC_10, group = package, color = NULL, fill = package)) +
ggplot2::geom_bar(stat = "identity", position = ggplot2::position_dodge()) +
ggplot2::facet_wrap(~ contrast) +
ggplot2::theme(axis.text.x = ggplot2::element_text(angle = 90, vjust = 0.5, hjust = 1))
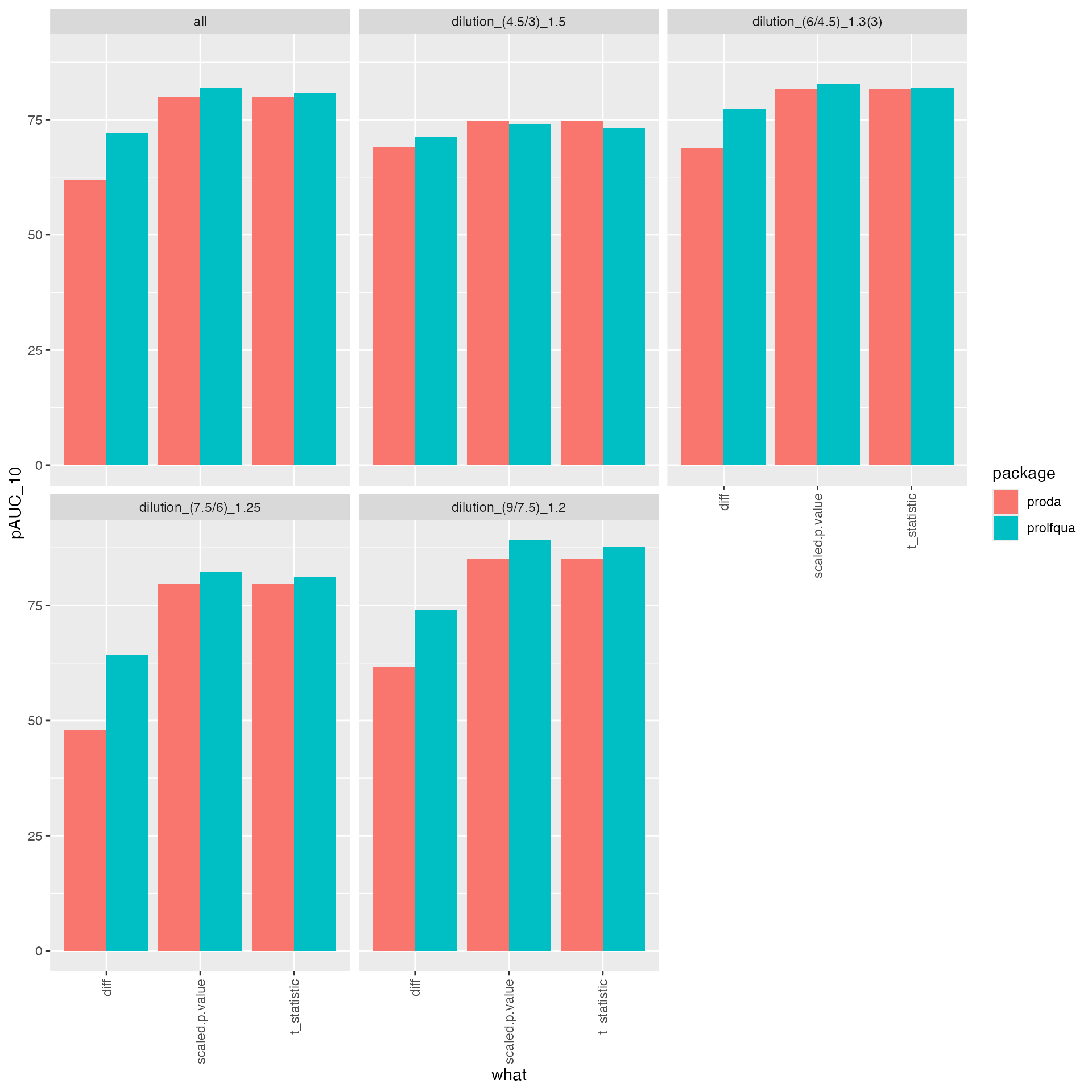